Welcome! And let's jump right into it
I'm starting a Substack and we're getting stuck in... immediately
Welcome!
As some of you know, my name is Arman Kafai. You may have seen my work on Twitter (I refuse to call it X) or stumbled upon my American soccer analysis on Backheeled, where I do most of my writing.
In my former life before re-entering the mediascape, I was a data consultant for FC Dallas, going from the 2021 season until the 2023 season. Working closely with the club gave me knowledge and an understanding of the sport from a completely new perspective, ranging from explaining concepts to first-team coaches to trying to solve problems throughout the season.
You’ve probably gotten this far and asked, “Arman, you have an outlet you write for. Why create a Substack?”
There are a lot of concepts that I love to explore and investigate further, that may not be American soccer-centric. Concepts about how we can translate what we see in our data to coaches and front office executives and how our beautiful game may evolve in future years. Topics like that struggle to find a home in my ventures, so why not explain to an audience on this wonderful site?
Enough about me. Let’s get right into it.
Taking a Page From Hockey: Analyzing Shot Danger by xG
During my days of working in soccer, there was a common question I was asked by multiple people, across multiple coaching staffs.
“Arman, what is a good xG on a shot?”
Often, I’d laugh and say, “Well the higher the xG, the better the shot!” and move on. After a while though, that answer just didn’t sit right with me. Often, you see goals with values that aren’t that high when it comes to xG. You’ll see a .30, a .25, the occasional .42, and so on.
In fact, in 2023, the xG with the most shots in MLS was .02 (1835). Next? .03 at 1648.
As you can tell… the distribution is not equal throughout (with a little blip at .79 for penalties). Wanting to dig deeper, I began looking into a sport that has embraced analytics and shares some similarities with soccer.
Hockey.
In hockey, they have embraced analytics in many ways. They have xG, xG for keepers, and many other metrics that mirror soccer. But the part that intrigued me was how they categorize xG chances.
Based on where a player takes a shot on the ice, the shot will be given a value of 1,2, or 3 and will be categorized as a high, medium, or low-danger shot.
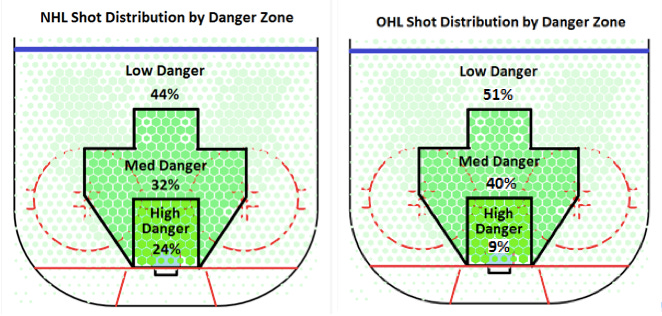
Within this, sites like MoneyPuck take those shots and pair them with expected goal values, as linked above. It seemed like a very simple thing to say and explain to coaches. Instead of talking about xG and what value a specific shot had during a match, I could create a concept like that for our post-match reports.
I never had the time to implement this during my time at the club but once I left, I had a devoid of time and dug into the concept. My plan: find the xG thresholds that made sense statistically, and categorize shots by high danger, medium danger, and low danger.
Except to my surprise, someone had already made my plan for me.
Enter Jamon Moore and Carlon Carpenter’s ‘Where Goals Come From’ series. In it, they detailed where goals came from on the field, how to train them with coaches, and more. To be honest, it is a must-read for anyone involved with soccer. While I was digging through their piece, this graphic that Jamon made popped up, using pre-shot xG.
You can read more about how Jamon came up with these thresholds here, but as you can see, based on historical trends, this is exactly what I envisioned. You now have four tiers where we can categorize shots and we have data to back it up, thanks to Jamon’s excellent work.
It’s why I have an issue at times when people just point out a team’s total xG versus digging deeper. It’s probably the former data consultant in me and yes, I understand at a higher level, we shouldn’t be looking at match to match xG as much. It’s fun though and coaches do ask for this information.
The deeper issue lies in a single shot accounting for a lot of the xG for the team. An example of this was pretty clear in last week’s Leagues Cup match between Austin FC and Pumas.
Austin FC defeated Pumas 3-2 in a crazy game. However, some people pointed to Pumas’ xG (while against 10 men for a lot of the match) and claimed that Austin’s result wasn’t great. As someone who watched the game, I didn’t get the same vibe, but the stats were pretty clear… right?
Shoutout to Sebastian (or as you may know him as
on Twitter) for compiling this information for me, but here’s how the xG broke down for both teams with our thresholds not including Pumas’ penalty.
Was Austin’s win unsustainable? They forced Pumas into taking 22 poor xG shots according to our thresholds above, which, according to the chart above, have about a 2% conversion rate. They allowed two shots of average xG, which resulted in around a 9% conversion rate; Pumas scored one of those chances. The one great xG chance Pumas had, they scored. Meanwhile, Austin had the same amount of great xG chances (and scored it through Driussi), and more good xG chances (and they scored that one too).
If you were to show this to a coach, I feel like they would look at this and be fine with it, especially the circumstances being down a man. That’s why I believe we may need to reapproach our way of analyzing matches for xG, compared to just looking at the total numbers and calling it a day. This more micro-level analysis is not only easier to digest for staff not well-versed in analytics, but it also makes sense to the common person.
It’s a bit more extra work for an analyst but once you’ve established a solid ground with your staff, I believe this would make reports or analysis of matches more digestable. Pair it with video and it’s even better.
This is only the beginning of an analysis like this. Can we pair PSxG with this threshold to create a matrix that says, hey great shot pre-shot, great shot post-shot that results in a goal (shoutout
for that idea)? What are these values for goalkeepers using PSxG? Is there an even better way to break this down?Share some comments and let me know what you think! I’m always open to discussion. Please shoot those mentioned a follow on their respective social media (as linked).
While I’m not sure how often I’ll be doing this, I’ll be posting it on my Twitter @ArmanKafai. Give that a follow if you please!
I think you have a very interesting proposal here. While it doesn’t change the mathematical interpretation of expected goals (the sum of expected values is still the expected value of the sum) I do think these ideas work towards a better use of data. If we can make the metrics less nuanced by bucketing them, I think we can move towards better informed coaching. If a team could trade 3 poor xG shots for one average xG shot, or 2 average xG shots for one good one, they will score more goals on fewer shots. The reverse is true for defense. Giving a discrete vocabulary to a continuous idea allows you to measure progress towards goals like this.
When I see a team like Austin play, especially how they played in the group stage of Leagues Cup this year, I have to think discussion like these are part of what is going on. Wolff’s defensive approach really seems aligned with this thinking. I’m hoping the new personnel will start making the approach work on offense. I haven’t been too disappointed with the goal scoring this season. It seems like a team that is being coached creatively in a way that will become more and more common in all leagues. I think there are efforts to innovate that are hard to appreciate with xG alone.
This is exactly the clarification and spotlight this stat needed. Far too often the stat is given too much weight without enough context. Really appreciate the insight and knowledge drop! We look forward to many more learning moments!